 |
|
|
|
|
Revenue management has traditionally been concerned with managing scarce capacity by using pricing mechanisms and demand management as an operational tool. The practice of revenue management is taking hold in many industries and takes various forms: capacity allocation controls, dynamic pricing, dynamic bundling, bargaining and negotiated pricing, customized pricing, assortment optimization, auctions, and so on. From industries such as airlines, hotels and rentals, these days we also see such practices in e-commerce, taxis, energy, railways, and road pricing. The rise of platforms is another new phenomenon. Recent trends point to an unprecedented level of control over the design, implementation, and operation of markets: more than ever before, we are able to engineer the platforms governing transactions among market participants. As a consequence, market operators or platforms can control a host of variables such as pricing, liquidity, visibility, information revelation, terms of trade, and transaction fees. On its part, given these variables, market participants often face complex problems when optimizing their own decisions. Operational study of these platforms and their design is still in their infancy and we encourage submissions studying these markets, both from the perspective of the market operator and the market participants.
We seek well-written papers that are connected to reality, improve our understanding of the application domain at-hand, and ideally have a possibility of implementation. We look for a mix of approaches including modeling, theoretical, empirical, or computational. The paper should score high on most of the following criteria: (1) How realistic and significant is the application studied?; (2) How original/creative is the approach adopted?; (3) How significant would be its impact on practice?
Associate Editors: Itai Ashlagi, Santiago Balseiro, Kostas Bimpikis, Rene Caldentey, Felipe Caro, Arnoud den Boer, Karan Girotra, Stefanus Jasin, Jun Li, Ilan Lobel, Brendan Lucier, Vahideh Manshadi, Marcelo Olivares, Saša Pekeč, Sridhar Seshadri, Huseyin Topaloglu, Senthil Veeraraghavan, Fanyin Zheng. |

|
|
|
|
|
The Department of Services, Platforms, and Revenue Management seeks manuscripts that offer enduring knowledge to improve our understanding of the design, performance, and their key drivers of service systems, platform management, and revenue management. The services or platforms of interest could involve interactions among businesses and/or consumers, or be internal to an organization.
We value novel research questions, impactful contributions, and rigorous execution. A strong motivation derived from real world applications is necessary, along with a clear contribution to knowledge and/or a demonstration of managerial relevance. We embrace a variety of methods, including analytical modeling, empirical methods, lab and field experiments, and numerical methods (simulation, machine learning). Given the cross-functional nature of services, we are very much open to interdisciplinary contributions that use or integrate methods and/or knowledge from different fields (e.g., social sciences and engineering).
Industries of interest could range from physical to information-based services, the decisions could range from strategic to operational, and the scope of analysis could range from macroscopic (e.g., industries, organizations) to meso and microscopic (e.g., teams, individuals). Topics include: wait time and capacity management, pricing and revenue management, service contracting, digital platform management and scaling, marketplaces, e- and m-commerce, process design and digitalization, service quality management, servicization and productization, access and location, network management, customer/employee experience management, people operations and organizational design, service design and innovation, peer-to-peer services, crowdsourcing, service globalization, sustainable and/or ethical issues in services.
Relative to the other departments for which there is potential overlap (e.g., healthcare, analytics, innovation), we value contributions that are more practice-driven than methodological and that are generalizable beyond a single domain of application.
|

|
|
|
|
|
|
|
|
|
|
|
|
|
|
|
|
|
The Revenue Management and Market Analytics area considers articles at the forefront of advancing the theory and practice of pricing and revenue optimization, and design and operation of market platforms.
Rapid changes in information, communication, and computing technology that are altering all aspects of our economic interactions. Not only do these advances enable us to collect ever more detailed data on customer and firm behavior, but they also enable much finer grained optimization of all the determinants of market outcomes. As a consequence, market operators or platforms can control a host of variables such as pricing, liquidity, search and matching, information revelation, terms of trade, and transaction fees. Analogously, market participants have far greater control over bidding, pricing, assortment optimization, and revenue optimization. These forces are being borne out in the rise of online marketplaces across a wide range of industries: more than ever before, we are able to engineer the platforms governing transactions among market participants.
These forces are evident in the spectrum of academic research pushing beyond traditional models of pricing and revenue management: more sophisticated models of consumer choice and behavioral biases; real-time pricing decisions (e.g., as in ride-sharing and ad auctions); fine-grained, dynamic search and matching algorithms; novel rating and review systems; dynamic bundling (and unbundling) of products; personalized assortments and discounts; etc.
We seek high-impact papers with deep roots in real problems, with a slight preference for (but not limited to) significant methodological or analytical contributions. We also welcome papers that excel in their modeling and/or computational approach to solving a relevant practical problem, supported by a well-documented numerical study, ideally based on real data.
Associate Editors: Omar Besbes, Kostas Bimpikis, Rene Caldentey, William Cooper, Jose Correa, Vivek Farias, Vineet Goyal, Ming Hu, Nicole Immorlica, Srikanth Jagabathula, Yash Kanoria, Ilan Lobel, Paat Rusmevichientong, and Huseyin Topaloglu.
|
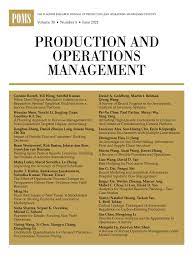 |
|
|
|
|
|