Dividing and Conquering Big Data
The TRIDENT Method for Partitioning and Synthesizing Advanced Analytics
Speaker:
Dan Steinberg, Chief Scientist and Product Evangelist
Salford Systems (a Minitab Company)
Co-Author: Nicholas Scott Cardell
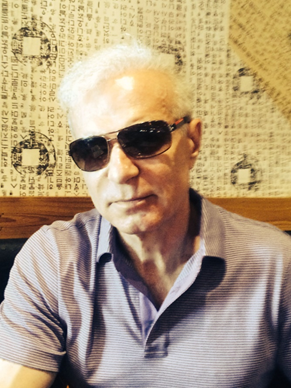
When: Wednesday, October 10, 2018, 12 Noon – 2PM
Place: The Penn Club,
Between 5th and 6th Avenues in Midtown Manhattan
Abstract:
Breaking large problems into smaller subproblems has a long and illustrious history in data analytics. To date the way such problems are partitioned has been ad-hoc and undisciplined with random partitioning being the most common method. We introduce TRIDENT, a structured methodology which has roots in experimental design theory, that allows analysts to construct ensembles of machine learning models exhibiting highly desirable statistical properties and supports the development of superior ensembles of ensembles. When applied to the rows of a data table TRIDENT looks like a form of cross-validation and when applied to the columns (predictors) it offers a novel approach to variable selection that can take the simultaneous relevance of a group of predictors into account.
The name “Trident” is inspired by the three prongs making up the methodology.
1: Trident uses a new Cross-Validation (CV) scheme that has better properties than conventional CV.
Trident uses Galois number theory to construct the parts and folds of a cross-validation resulting in plans that can be described as extensions to Latin Squares or Latin Hyper Cube based experimental design. In standard cross validation there is no overlap in the data excluded (test data) from any pair of folds. We thus have no way to tell what portion of the total variance of our predictions is due to the signal versus variance in the training data versus variance in the learning process itself. Trident always has an overlap between the data excluded from any two folds and this can be leveraged to great advantage.
Prong 2: A new predictive model (estimator).
The new Trident predictive model is an ensemble consisting of all the models developed in the folds. Specifically, the new estimator is a calibrated average of the fold-specific CV models.
An important advance of the TRIDENT ensemble is that each of the component models is synchronized to exploit the fact that the solo models will subsequently be part of an ensemble. The component models are intentionally “overtrained” or “overfit” understanding that the averaging inherent in the ensembling will eliminate the excess noise that would otherwise harm a solo overfit model. This synchronized “overfitting” is an innovation that allows us to extract more signal from data than has hitherto been possible with either solo models or ad-hoc unplanned ensembles.
Prong 3: Better estimates of the statistical properties of both the new
estimator and the original estimator. Our discussion illustrates this with reference to the gradient boosting machine (GBM).
Speaker Bio:
Dan Steinberg, Ph.D. Harvard University (Econometrics) was the founder of Salford Systems, one of the world’s first software companies in the field of machine learning. Working closely with Leo Breiman and Jerome Friedman since 1990 Salford introduced commercial software based on Friedman’s proprietary code for the CART decision tree, MARS regression splines, and the first gradient boosting machine (TreeNet) and Breiman and Cutler’s Random Forests. Dan led the teams that won the KDDCup 2000 predictive modeling competition and also the 2002 Teradata/Duke Churn modeling competition and was involved in a number of other subsequent competition winning efforts. Besides software development Dan has been involved in major consulting projects for some of the world’s largest banks and has published in economics, statistics, and computer science journals.