When
May 18, 2016 | 12:00pm - 2:00pm
TOPIC
Random Forest versus Logistic Regression
ABSTRACT
Different methods generate different variable selection results, reflecting the needs for a comprehensive literature review. These different methods include correlation with dependent variable, p-value, information value, as well as variable importance, and their validities under different conditions.
Default option for Random Forest is biased towards continuous variables, less favor of categorical and binary variables. Unbiased solution is very complex and computationally intensive. There are opportunity to apply random forest variable importance to generate non-linear relationship, scaling, and interaction items to improve logistic regressions.
Speaker
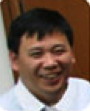 |
Yulin Ning
Senior Director, Citigroup Global Decision Management
Yulin Ning is a Senior Director in Global Decision Management, a global strategy and analytic division in Citigroup's Global Consumer Bank. His current focus is on next generation analytics which include behavior, unstructured data, digital, big data, and advanced predictive analytics and machine learning. In his current role, he is responsible for utilizing emerging technology, tools, and advanced analytics to build creative business solutions. Yulin holds a Ph.D. in Agricultural Economics and he is a frequent speaker in the areas of "Emerging Analytics". Yulin had the experience to lead a CCAR modeling team and is an early adaptor of digital analytical, machine learning, and big data from next generation analytic perspectives.
|